Predictive Analytics involves use of quantitative analysis, statistical algorithms and historical data to make projections about the future. It adds value in business as it assists them to make the right decisions and manage resources appropriately in relation to risk management.
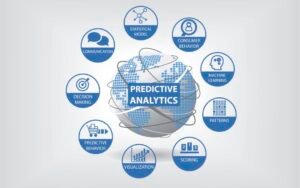
Unleashing the Power of Predictive Analytics with OneStream
The Challenge Multinational Corporations Face
Key forecasting issues that large MNCs face include unpredictability. Complex data, lack of centralized data organization, and widespread regional differences, as well as paper-based processes, result in low efficiency, missed opportunities, and bad decisions. Factors such as market growth, changes, and seasonal variations make forecasting even more unpredictable, requiring a more efficient and precise approach from MNCs.
The Goal: Data-Driven Transformation
One such MNC, ABC, embarked on a journey to revolutionize its forecasting approach using Predictive Analytics 123 by OneStream. The primary objectives were:
- Enhancing Forecast Accuracy: Leveraging advanced models like SARIMA and Holt-Winters to better capture trends and seasonality.
- Centralizing Forecasting: Unifying operations across all regions on a single, automated platform.
- Improving Efficiency: Automating manual processes to save time and resources.
- Driving Data-Driven Decisions: Using accurate forecasts to optimize inventory, cash flow, and budgeting.
Roadmap to Predictive Excellence
ABC’s roadmap for implementing Predictive Analytics 123 included the following steps:
- Setup and Installation:
- Installed the Predictive Analytics solution from the OneStream Marketplace.
- Configured application server settings and assigned security roles.
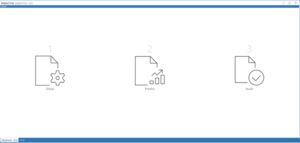
- Data Preparation:
- Gathered three years of clean historical data to predict growth for the next 12 months.
- Defined data ranges to ensure accuracy.
- Template and Cube View Creation:
- Set up forecasting templates with source and target scenarios.
- Assigned cube views and selected models like ARIMA and Holt-Winters based on data characteristics.
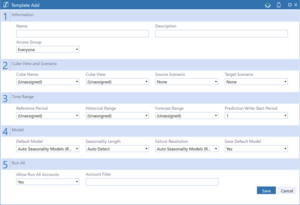
- Model Execution and Adjustment:
- Ran predictive models and reviewed accuracy using metrics like MAPE and MPE.
- Refined the models based on test outcomes to enhance precision.
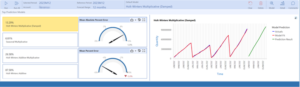
- Scenario Planning:
- Simulated business conditions (e.g., market fluctuations) to prepare for potential impacts.
- Made adjustments to forecasts to ensure adaptability.
- Implementation and Monitoring:
- Integrated forecasts into decision-making processes such as budgeting and inventory management.
- Continuously monitored model performance and made necessary improvements.
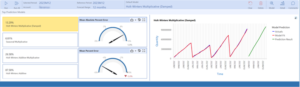
Forecasting Methods: The Backbone of Predictive Analytics
Predictive Analytics 123 offers several robust forecasting methods to suit various data patterns:
- Simple Exponential Smoothing: A method for datasets without trends or seasonality, using a single parameter to control influence.
- Holt-Winters: Extends exponential smoothing by accounting for both trends and seasonality.
- SARIMA: Adds seasonal components to ARIMA, effectively handling recurring cycles in data.
By combining these models, ABC could achieve accurate and reliable predictions for even the most complex datasets.